“Welcome aboard to BigCo!”
“Thanks! I’m excited to be here. This is my first tech job, even if it is just an internship.”
“We’re going to start you off with some automated testing. You’re familiar with queues, right?”
“The data structure? Sure thing. First in, first out.”
“Great! We need some help validating that our queueing module is always working properly. We have a bunch of test scenarios written, and we want need to someone to check that the observed behavior of the queue is correct.”
“So, for input, do I get something like a history of interactions with the queue? Like this?”
q.add("A") -> OK
q.add("B") -> OK
q.pop() -> "A"
q.add("C") -> OK
q.pop() -> "B"
q.pop() -> "C"
“Exactly! That’s a nice example of a correct history for a queue. Can you write a program that takes a history like that as input and returns true if it’s a valid history?”
“Sure thing.”
“Excellent. We’ll also need your help generating new test scenarios.”
A few days later
“I think I found a scenario where the queue is behaving incorrectly when it’s called by a multithreaded application. I got a behavior that looks like this:”
q.add("A") -> OK
q.add("B") -> OK
q.add("C") -> OK
q.pop() -> "A"
q.pop() -> "C"
q.pop() -> "B"
“Hmmm. That’s definitely incorrect behavior. Can you show me the code you used to generate the behavior?”
“Sure thing. I add the elements to the queue in one thread, and then I spawn a bunch of new threads and dequeue in the new threads. I’m using the Python bindings to call the queue. My program looks like this.”
from bigco import Queue
from threading import Thread
def pop_and_print(q):
val = q.pop()
print(val)
q = Queue()
q.add("a")
q.add("b")
q.add("c")
Thread(target=pop_and_print, args=[q]).run()
Thread(target=pop_and_print, args=[q]).run()
Thread(target=pop_and_print, args=[q]).run()
“And the output looked like this:”
A
C
B
“Well, that’s certainly not the order I expect the output to be printed in, but how do you know the problem is that the queue is actually behaving correctly? It might be that the values were dequeued in the correct order, but because of the way the threads are scheduled, the print statements were simply executed in a different order than you expect.”
“Hmmm. I guess you’re right: just looking at the order of the printed output doesn’t give me enough information to tell if the queue is behaving correctly or not. Let me try printing out the thread ids and the timestamps.”
[id0] [t=1] before pop
[id0] [t=2] after pop
[id0] [t=3] output: A
[id1] [t=4] before pop
[id2] [t=5] before pop
[id2] [t=6] after pop
[id2] [t=7] output: C
[id1] [t=8] after pop
[id1] [t=9] output: B
“Oh, I see what happened! The operations of thread 1 and thread 2 were interleaved! I didn’t think about what might happen in that case. It must have been something like this:”
[id0] [id1] [id2]
q.pop()->"A"
print("A")
q.pop()->"B"
q.pop()->"C"
print("C")
print("B")
“Well, it looks like the behavior is still correct, the items got dequeued in the expected order, it’s just that they got printed out in a different order.”
The next day
“After thinking through some more multithreaded scenarios, I ran into a weird situation that I didn’t expect. It’s possible that the “pop” operations overlap in time across the two different threads. For example, “pop” might start on thread 1, and then in the middle of the pop operation, the operating system schedules thread 2, and it starts in the middle.”
[id0] [id1] [id2]
q.pop(): start
q.pop(): end
print("A")
q.pop(): start
| q.pop(): start
q.pop(): end |
q.pop(): end
print("C")
print("B")
“Let’s think about this. If id1 and id2 overlap in time like this, what do you think the correct output should be? ‘ABC’ or ‘ACB’?”
“I have no idea. I guess we can’t say anything!”
“So, if the output was ‘ABB’, you’d consider that valid?”
“Wait, no… It can’t be anything. It seems like either ‘ABC’ or ‘ACB’ should be valid, but not “ABB”.
“How about ‘BCA’? Would that be valid here?”
“No, I don’t think so. There’s no overlap between the first pop operation and the others, so it feels like the pop in id0 should return “A”.
“Right, that makes sense. So, in a concurrent world, we have potentially overlapping operations, and that program you wrote that checks queue behaviors doesn’t have any notion of overlap in it. So we need to be able to translate these potentially overlapping histories into the kind of sequential history your program can handle. Based on this conversation, we can use two rules:
1. If two operations don’t overlap (like the pop in id0 and the pop in id1) in time, then we use the time ordering (id0 happened before id1).
2. If two operations do overlap in time, then either ordering is valid.
“So, that means that when I check whether a multithreaded behavior is valid, I need to actually know the time overlap of the operations, and then generate multiple possible sequential behaviors, and check to see if the behavior that I witnesses corresponds to one of those?”
“Yes, exactly. This is a consistency model called linearizability. If our queue has linearizable consistency, that means that for any behavior you witness, you can define a linearization, an equivalent sequential behavior. Here’s an example.”
[id0] [id1] [id2]
q.add("a")
q.add("b")
q.add("c")
q.pop(): start
q.pop()->"A"
q.pop(): start
| q.pop(): start
| q.pop()->"C"
q.pop()->"B"
“The question is: can we generate a linearization based on the two rules above? We can! Because the “id1” and “id2” overlap, we can generate a linearization where the “id1″ operation happens first. One way to think about it is to identify a point in time between the start and end of the operation and pretend that’s when the operation really happens. I’ll mark these points in time with an ‘x’ in the diagram.
[id0] [id1] [id2]
q.add("a")
q.add("b")
q.add("c")
q.pop(): start
x
q.pop()->"A"
q.pop(): start
q.pop(): start
x
x
q.pop()->"C"
q.pop()->"B"
“Now we can rewrite this as a linear history.”
q.add("a")
q.add("b")
q.add("c")
q.pop()->"A"
q.pop()->"B"
q.pop()->"C"
Going distributed
“We’re expanding our market. We’re building on our queue technology to build a distributed queue. We’re also providing a new operation: “get”. When you call “get” on a distributed queue, you get the entire contents of the queue, in queue order.”
“Oh, so a valid history would be something like this?”
q.add("A")
q.add("B")
q.get() -> ["A","B"]
q.add("C")
q.get() -> [A","B","C"]
“Exactly! One use case we’re targeting is using our queue for implementing online chat, so the contents of a queue might look like this:”
["Alice: How are you doing?",
"Bob: I'm fine, Alice. How are you?",
"Alice: I'm doing well, thank you."]
CAPd
“OK, I did some testing with the distributed queue. ran into a problem with the distributed queue. Look at this history, it’s definitely wrong. Note that the ids here are process ids, not thread ids, because we’re running on different machines.
[id0] [id1]
q.add("Alice: Hello"): start
q.add(...) -> OK
q.add("Bob: "Hi"): start
q.add(...)->OK
q.get(): start
q.get()-> ["Bob: Hi"]
“When process 1 called ‘get’, it didn’t see the “Alice: Hello” entry, and that operation completed before the ‘get’ started! This history isn’t linearizable!”
“You’re right, our distributed queue isn’t linearizable. Note that we could modify this history to make it linearizable if process 0’s add operation did not complete until after the get:
[id0] [id1]
q.add("Alice: Hello"): start
q.add("Bob: "Hi"): start
q.add(...) -> OK
q.get(): start
q.get()-> ["Bob: Hi"]
q.add(...) -> OK
“Now we can produce a valid linearization from the history”
q.add("Bob: "Hi")
q.get()->["Bob: Hi"]
q.add("Alice: Hello")
“But look what we had to do: we had to delay the completion of that add operation. This is the lesson of the CAP theorem: if you want your distributed object to have linearizable consistency, then some operations might take an arbitrarily long time to complete. With our queue, we decided to prefer availability, so that all operations are guaranteed to complete within a certain period of time. Unfortunately, once we give up on linearizability, things can get pretty weird. Let’s see how many different types of weird things you can find.”
Monotonic reads
“Here’s a weird one. The ‘Hi’ message disappeared in the second read!”
[id0] [id1] [id2]
q.add("A: Hello")
q.add("B: Hi")
q.get()->["A: Hello", "B: Hi"]
q.get()->["A: Hello"]
“Yep, this violates a property called monotonic reads. Once process 0 has seen the effect of the add(“B: Hi”) operation, we expect that it will always see it in the future. This is an example of a session property. If the two gets happened on two different processes, this would not violate the monotonic reads property. For example, the following history doesn’t violate monotonic reads, even though the operations and ordering are the same. That’s because one of the gets is in process 0, and the other is in process 1, and the monotonic reads property only applies to reads within the same process.
[id0] [id1] [id2]
q.add("A: Hello")
q.add("B: Hi")
q.get()->["A: Hello", "B: Hi"]
q.get()->["A: Hello"]
“All right, let’s say we can guarantee monotonic reads. What other kinds of weirdness happen?”
Read your writes
[id0]
q.add("A: Hello")
q.get() -> []
“Read your writes is one of the more intuitive consistency properties. If a process writes data, and then does a read, it should be able to see the effective of the write. Here we did a write, but we didn’t see it.”
Writes follow reads
[id0]
q.get() -> []
q.get() -> ["A: Hello"]
q.add("A: Hello")
“Here’s a case where read-your-writes isn’t violated (in fact, we don’t do any reads after the write), but something very strange has happened. We saw the effect of our write before we actually did the write! This violates the writes follow reads property. This also called session causality, and you can see why: when it was violated, we saw the effect before the cause!”
Monotonic writes
[id0] [id1]
q.add("A: Hi there!")
q.add("A: How are you?")
q.get() -> ["A: How are you?"]
“Hey, process 1 saw the ‘How are you?’ but not the ‘Hi there!’, even though they both came from process 0.”
“Yep. It’s weird that process 1 saw the second write from process 0, but it didn’t see the first write. This violates the monotonic writes property. Note that if the two writes were from different processes, this would not violate the property. For example, this would be fine:
[id0] [id1]
q.add("A: Hi there!")
q.add("A: How are you?")
q.get() -> ["A: How are you?"]
Consistent prefix
[id0] [id1]
q.add("A: Hello")
q.add("B: Hi")
q.get()->["B: Hi"]
q.get()->["A: Hello", "B: Hi"]
“From process 1’s perspective, it looks like the history of the chat log changed! Somehow, ‘A: Hello’ snuck in before ‘B: Hi’, even though process 1 had already seen ‘B: Hi’.”
“Yes, this violates a property called consistent prefix. Note that this is different from monotonic reads, which is not violated in this case. (Sadly, the Jepsen consistency page doesn’t have an entry for consistent prefix).
Reasoning about correctness in a distributed world
One way to think about what it means for a data structure implementation to be correct is to:
- Define what it means for a particular execution history to be correct
- Check that every possible execution history for the implementation satisfies this correctness criteria.
Step 2 requires doing a proof, because in general there are too many possible execution histories for us to check exhaustively. But, even if we don’t actually go ahead and do the formal proof, it’s still useful to think through step 1: what it means for a particular execution history to be correct.
As we move from sequential data structures to concurrent (multithreaded) ones and then distributed ones, things get a bit more complicated.
Recall that for the concurrent case, in order to check that a particular execution history was correct, we had to see if we could come up with a linearization. We had to try and identify specific points in time when operations took effect to come up with a sequential version of the history that met our sequential correctness criteria.
In Principles of Eventual Consistency, Sebastian Burckhardt proposed a similar type of approach for validating the execution history of a distributed data structure. (This is the approach that Viotti & Vukolic extended. Kyle Kingsbury references Viotti and Vukolic on the Jepsen consistency models page that I’ve linked to several times here).
Execution histories as a set of events
To understand Burckhardt’s approach, we first have to understand how he models a distributed data structure execution history. He models an execution history as a set of events, where each event has associated with it:
- The operation (including arguments), e.g.:
- get()
- add(“Hi”)
- A return value, e.g.
- [“Hi”, “Hello”]
- OK
He also defines two relations on these events, returns-before and same-session.
Returns-before
The returns-before (rb) relation models time. If there are two events, e1, e2, and (e1,e2) is in rb, that means that the operation associated with e1 returned before the operation associated with e2 started.
Let’s take this example, where the two add operations overlap in time:
[id0] [id1] [id2]
add("A: Hello"):start
| add("B: Hi"):start
| add("B: Hi"):end
add("A: Hello"):end
get()->["A: Hello", "B: Hi"]
get()->["A: Hello"]
I’ll use the following labeling for the events:
- e1: add(“A: Hello”)
- e2: add(“B: Hi”)
- e3: get() -> [“A: Hello”, “B:Hi”]
- e4: get() -> [“A: Hello”]
Here, rb={(e1,e3), (e1,e4),(e2,e3),(e2,e4),(e3,e4)}
Note that neither (e1,e2) nor (e2,e1) is in rb, because the two operations overlap in time. Neither one happens before the other.
Same-session
The same-session (ss) relation models the grouping of operations into processes. In the example above, there are three sessions (id0, id1, id2), and the same-session relation looks like this: ss={(e1,e1),(e1,e4),(e4,e1),(e4,e4),(e2,e2),(e3,e3)}. (Note: in this case, there are only two operations that are in the same session, e1 and e4
This is what the graph looks like with the returns-before (rb) and same-session (ss) relationship shown.
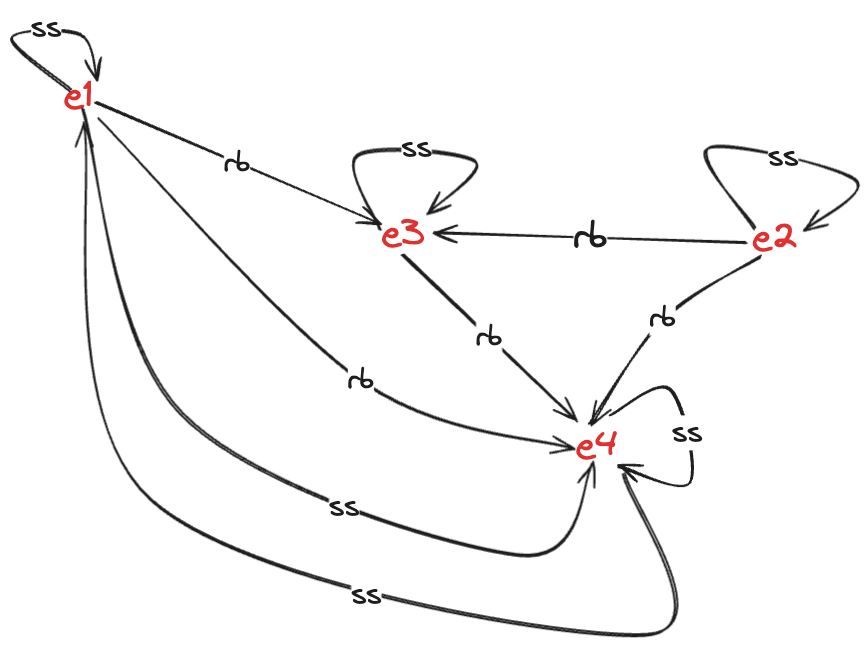
Explaining executions with visibility and arbitration
Here’s the idea behind Burckhardt’s approach. He defines consistency properties in terms of the returns-before (rb) relation, the same-session (ss) relation, and two other binary relations called visibility (vis) and arbitration (ar).
For example, an execution history satisfies read my writes if: (rb ∩ ss) ⊆ vis
In this scheme, an execution history is correct if we can come up with visibility and arbitration relations for the execution such that:
- All of the consistency properties we care about are satisfied by our visibility and arbitration relations.
- Our visibility and arbitration relations don’t violate any of our intuitions about causality.
You can think of coming up with visibility and arbitration relations for a history as coming up with an explanation for how the history makes sense. It’s a generalization of the process we used for linearizability where we picked a specific point in time where the operation took effect.
(1) tells us that we have to pick the right vis and ar (i.e., we have to pick a good explanation). (2) tells us that we don’t have complete freedom in picking vis and ar (i.e., our explanations have to make intuitive sense to human beings).
You can think of the visibility relation as capturing which write operations were visible to a read, and the arbitration relation as capturing how the data structure should reconcile conflicting writes.
Specifying behavior based on visibility and arbitration
Unfortunately, in a distributed world, we can no longer use the sequential specification for determining correct behavior. In the sequential world, writes are always totally ordered, but in the distributed world, we might have to deal with two different writes that aren’t ordered in a meaningful way.
For example, consider the following behavior:
[id0] [id1] [id2]
e1. add("A")
e2. add("B")
e3. get()->???
What’s a valid value for ???. Let’s assume we’ve been told that: vis={(e1,e3),(e2,e3)}. This means that both writes are visible to process 3.
Based on our idea of how this data structure should work, e3 should either be: [“A”,”B”] or [“B”,”A”]. But the visibility relationship doesn’t provide enough information to tell us which one of these it was. We need some additional information to determine what the behavior should be.
This is where the arbitration relation comes in. This relation is always a total ordering. (For example, if ar specifies an ordering of e1->e2->e3, then the relation would be {(e1,e2),(e1,e3),(e2,e3)}. ).
If we define the behavior of our distributed queue such that the writes should happen in arbitration order, and we set ar=e1->e2->e3, then e3 would have to be get()->[“A”,”B”].
Let’s look at a few examples:
[id0] [id1]
e1. add("A")
e2. add("B")
e3. get()->["B","A"]
e4. get()->["B","A"]
The above history is valid, we can choose: vis={(e1,e3),(e2,e3),(e1,e4),(e2,e4)} and ar=e2->e1->e3->e4
[id0] [id1]
e1. add("A")
e2. add("B")
e3. get()->["A","B"]
e4. get()->["B","A"]
The above history is invalid, because there’s no arbitration and visibility relations we can come up with that can explain both e3 and e4.
[id0] [id1]
e1. add("A")
e2. add("B")
e3. get()->["A"]
e4. get()->["B","A"]
The above history is valid, because we can do: vis={(e1,e3),(e2,e4),(e3,e4))}, ar=e1->e2->e3->e4. Note that even though (e2,e3) is in ar, e2 is not visible to e3, and an operation only has to reflect the visible writes.
People don’t like it when you violate causality
Remember the example from “writes follow reads”?
[id0]
e1. q.get() -> []
e2. q.get() -> ["A: Hello"]
e3. q.add("A: Hello")
Note that we can come up with valid vis and ar relations for this history:
- vis = {(e3,e2)}
- ar = e1->e3->e2
But, despite the fact that we can come up with an explanation for this history, it doesn’t make sense to us, because e3 happened after e2. You can see why this is also referred to as session causality, because it violates our sense of causality: we read a write that happened in the future!
This is a great example of one of the differences between programming and formal modeling. It’s impossible to write a non-causal program (i.e., a program whose current output depends on future inputs). On the other hand, in formal modeling, we have no such restrictions, so we can always propose “impossible to actually happen in practice” behaviors to look at. So we often have to place additional constraints on the behaviors we generate with formal models to ensure that they’re actually realizable.
Sometimes we do encounter systems that record history in the wrong order, which makes the history look non-causal.
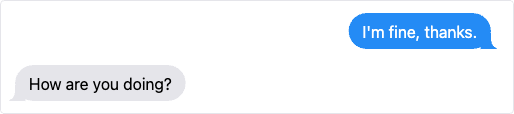
Consistency as constraints on relations
The elegant thing about this relation-based model of execution histories is that the consistency models can be expressed in terms of them. Burckhardt conveniently defines two more relationships.
Session-order (so) is the ordering of events within each session, expressed as: so = rb ∩ ss
Happens-before (hb) is a causal ordering, in the sense of Lamport’s Time, Clocks, and the Ordering of Events in a Distributed System paper. (e1,e2) is in hb if (e1,e2) is in so (i.e., e1 comes before e2 in the same session), or if (e1,e2) is in vis (i.e., e1 is visible to e2), or if there’s some transitive relationship (e.g., there’s some e3 such that (e1,e3) and (e3,e2) are in so or vis.
Therefore, happens-before is the transitive closure of so ∪ vis, which we write as: hb = (so ∪ vis)⁺ . We can define no circular causality as no cycles in the hb relation or, as Burckhardt writes it: NoCircularCausality = acyclic(hb)
If you made it all of the way here, I’d encourage you to check out Burckhardt’s Principles of Eventual Consistency book. You can get the PDF for free by clicking the “Publication” button the web page.
You might really enjoy Convex’s take on Serializability – the transactional corollary to Linearizability (at least that’s how I think about it): https://docs.convex.dev/database/advanced/occ
And more academic background (old but still relevant): https://www.usenix.org/conference/atc12/technical-sessions/presentation/cowling